Artificial Intelligence
Artificial intelligence (AI) systems already greatly impact our lives — they increasingly shape what we see, believe, and do. Based on the steady advances in AI technology and the significant recent increases in investment, we should expect AI technology to become even more powerful and impactful in the following years and decades.
It is easy to underestimate how much the world can change within a lifetime, so it is worth taking seriously what those who work on AI expect for the future. Many AI experts believe there is a real chance that human-level artificial intelligence will be developed within the following decades, and some think it will exist much sooner.
How such powerful AI systems are built and used will be very important for the future of our world and our own lives. All technologies have positive and negative consequences, but with AI, the range of these consequences is extraordinarily large: the technology has immense potential for good. Still, it comes with significant downsides and high risks.
A technology that has such an enormous impact needs to be of central interest to people across our entire society. But currently, the question of how this technology will get developed and used is left to a small group of entrepreneurs and engineers.
With our publications on artificial intelligence, we want to help change this status quo and support a broader societal engagement.
On this page, you will find key insights, articles, and charts of AI-related metrics that let you monitor what is happening and where we might be heading. We hope that this work will be helpful for the growing and necessary public conversation on AI.
Research & Writing
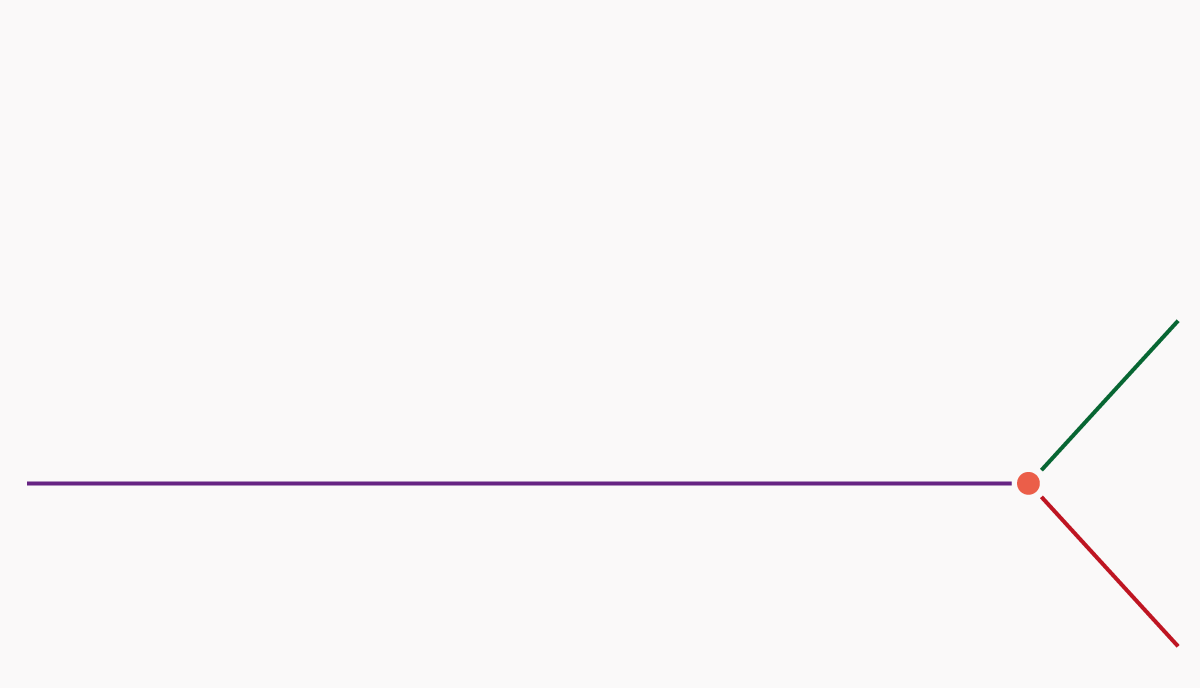
Artificial intelligence is transforming our world — it is on all of us to make sure that it goes well
How AI gets built is currently decided by a small group of technologists. As this technology is transforming our lives, it should be in all of our interest to become informed and engaged.
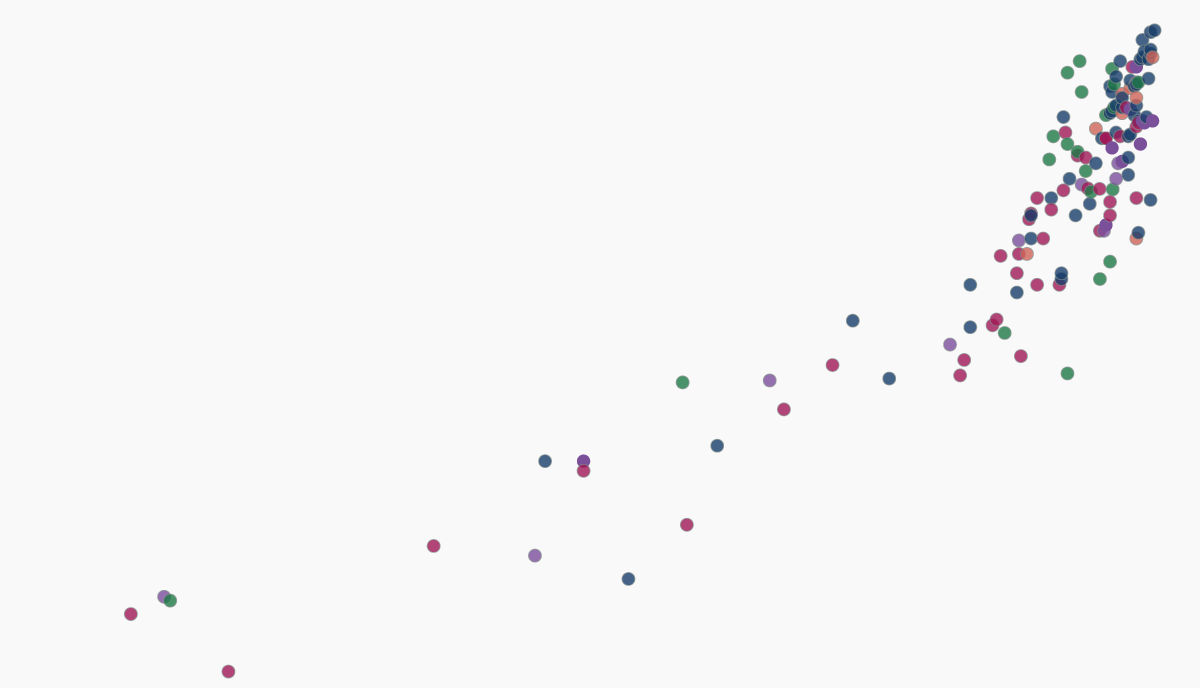
The brief history of artificial intelligence: The world has changed fast – what might be next?
Despite their brief history, computers and AI have fundamentally changed what we see, what we know, and what we do. Little is as important for the future of the world, and our own lives, as how this history continues.
More Articles on Artificial Intelligence
Artificial intelligence has advanced despite having few resources dedicated to its development – now investments have increased substantially
AI timelines: What do experts in artificial intelligence expect for the future?
Technology over the long run: zoom out to see how dramatically the world can change within a lifetime
Endnotes
We write “hypothetical” because no single person could perform this well across such varied tests. The authors based their analysis on expert performance on a subset of the tests for which there is human performance data – with “experts” considered to have the 95th percentile scores – and imagined a hypothetical person who would perform at this very high level across all tasks.
Hendrycks, Dan, et al. "Measuring massive multitask language understanding." arXiv preprint arXiv:2009.03300 (2020). https://arxiv.org/abs/2009.03300
Cite this work
Our articles and data visualizations rely on work from many different people and organizations. When citing this topic page, please also cite the underlying data sources. This topic page can be cited as:
Charlie Giattino, Edouard Mathieu, Veronika Samborska and Max Roser (2023) - “Artificial Intelligence” Published online at OurWorldinData.org. Retrieved from: 'https://ourworldindata.org/artificial-intelligence' [Online Resource]
BibTeX citation
@article{owid-artificial-intelligence,
author = {Charlie Giattino and Edouard Mathieu and Veronika Samborska and Max Roser},
title = {Artificial Intelligence},
journal = {Our World in Data},
year = {2023},
note = {https://ourworldindata.org/artificial-intelligence}
}
Reuse this work freely
All visualizations, data, and code produced by Our World in Data are completely open access under the Creative Commons BY license. You have the permission to use, distribute, and reproduce these in any medium, provided the source and authors are credited.
The data produced by third parties and made available by Our World in Data is subject to the license terms from the original third-party authors. We will always indicate the original source of the data in our documentation, so you should always check the license of any such third-party data before use and redistribution.
All of our charts can be embedded in any site.