AI timelines: What do experts in artificial intelligence expect for the future?
Many AI experts believe there is a real chance that human-level artificial intelligence will be developed within the next decades, and some believe that it will exist much sooner.
Artificial intelligence (AI) that surpasses our own intelligence sounds like the stuff from science-fiction books or films. What do experts in the field of AI research think about such scenarios? Do they dismiss these ideas as fantasy, or are they taking such prospects seriously?
A human-level AI would be a machine, or a network of machines, capable of carrying out the same range of tasks that we humans are capable of. It would be a machine that is “able to learn to do anything that a human can do”, as Norvig and Russell put it in their textbook on AI.1
It would be able to choose actions that allow the machine to achieve its goals and then carry out those actions. It would be able to do the work of a translator, a doctor, an illustrator, a teacher, a therapist, a driver, or the work of an investor.
In recent years, several research teams contacted AI experts and asked them about their expectations for the future of machine intelligence. Such expert surveys are one of the pieces of information that we can rely on to form an idea of what the future of AI might look like.
The chart shows the answers of 352 experts. This is from the most recent study by Katja Grace and her colleagues, conducted in the summer of 2022.2
Experts were asked when they believe there is a 50% chance that human-level AI exists.3 Human-level AI was defined as unaided machines being able to accomplish every task better and more cheaply than human workers. More information about the study can be found in the fold-out box at the end of this text.4
Each vertical line in this chart represents the answer of one expert. The fact that there are such large differences in answers makes it clear that experts do not agree on how long it will take until such a system might be developed. A few believe that this level of technology will never be developed. Some think that it’s possible, but it will take a long time. And many believe that it will be developed within the next few decades.
As highlighted in the annotations, half of the experts gave a date before 2061, and 90% gave a date within the next 100 years.
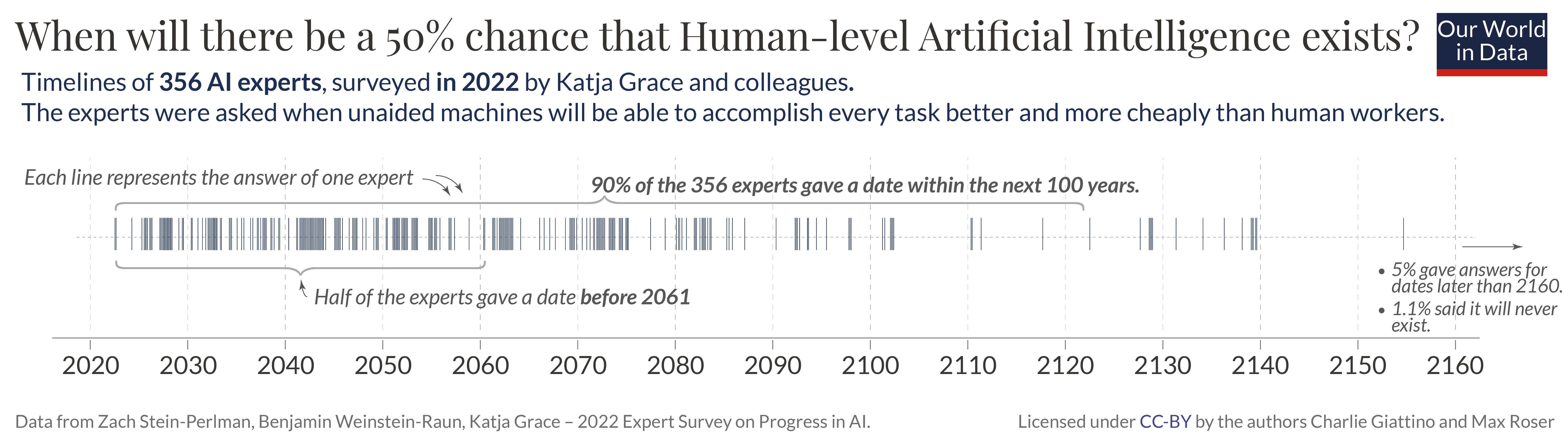
Other surveys of AI experts come to similar conclusions. In the following visualization, I have added the timelines from two earlier surveys conducted in 2018 and 2019. It is helpful to look at different surveys, as they differ in how they asked the question and how they defined human-level AI. You can find more details about these studies at the end of this text.
In all three surveys, we see a large disagreement between experts and they also express large uncertainties about their own individual forecasts.5
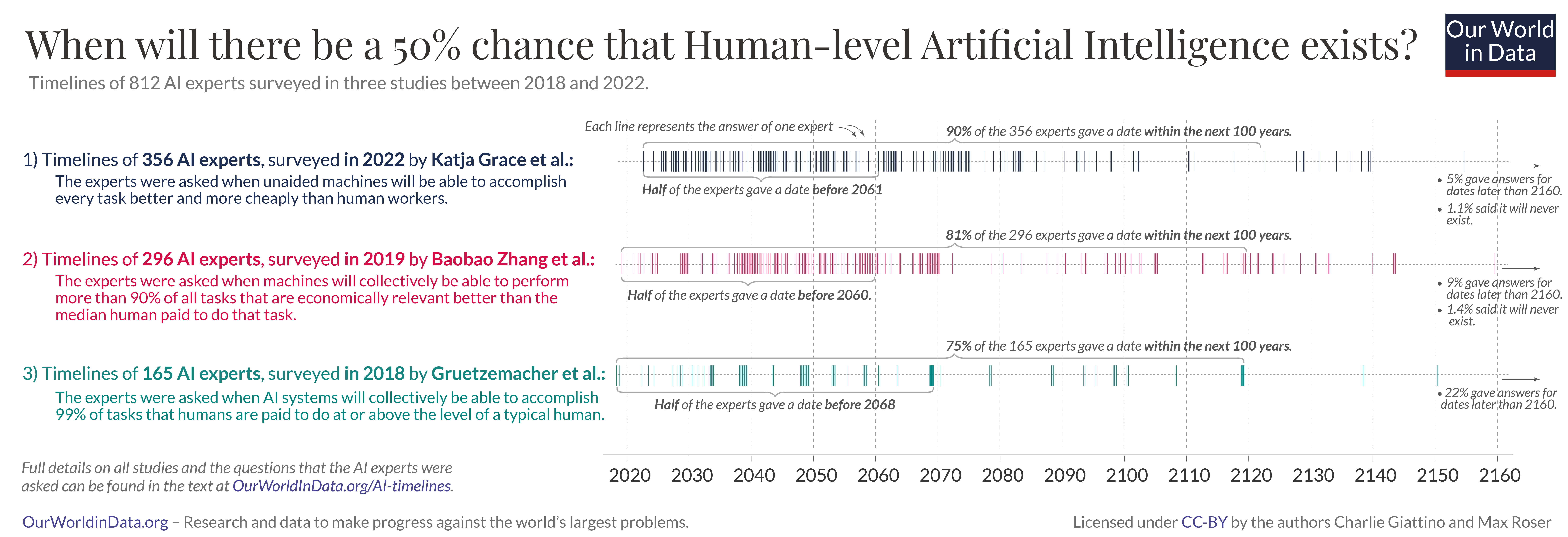
What should we make of the timelines of AI experts?
Expert surveys are one piece of information to consider when we think about the future of AI, but we should not overstate the results of these surveys. Experts in a particular technology are not necessarily experts in making predictions about the future of that technology.
Experts in many fields do not have a good track record in making forecasts about their own field, as researchers including Barbara Mellers, Phil Tetlock, and others have shown.6 The history of flight includes a striking example of such failure. Wilbur Wright is quoted as saying, "I confess that in 1901, I said to my brother Orville that man would not fly for 50 years." Two years later, ‘man’ was not only flying, but it was these very men who achieved the feat.7
Additionally these studies often find large ‘framing effects’, two logically identical questions get answered in very different ways depending on how exactly the questions are worded.8
What I do take away from these surveys however, is that the majority of AI experts take the prospect of very powerful AI technology seriously. It is not the case that AI researchers dismiss extremely powerful AI as mere fantasy.
The huge majority thinks that in the coming decades there is an even chance that we will see AI technology which will have a transformative impact on our world. While some have long timelines, many think it is possible that we have very little time before these technologies arrive. Across the three surveys more than half think that there is a 50% chance that a human-level AI would be developed before some point in the 2060s, a time well within the lifetime of today’s young people.
The forecast of the Metaculus community
In the big visualization on AI timelines below, I have included the forecast by the Metaculus forecaster community.
The forecasters on the online platform Metaculus.com are not experts in AI but people who dedicate their energy to making good forecasts. Research on forecasting has documented that groups of people can assign surprisingly accurate probabilities to future events when given the right incentives and good feedback.9 To receive this feedback, the online community at Metaculus tracks how well they perform in their forecasts.
What does this group of forecasters expect for the future of AI?
At the time of writing, in November 2022, the forecasters believe that there is a 50/50-chance for an ‘Artificial General Intelligence’ to be ‘devised, tested, and publicly announced’ by the year 2040, less than 20 years from now.
On their page about this specific question, you can find the precise definition of the AI system in question, how the timeline of their forecasts has changed, and the arguments of individual forecasters for how they arrived at their predictions.10
The timelines of the Metaculus community have become much shorter recently. The expected timelines have shortened by about a decade in the spring of 2022, when several impressive AI breakthroughs happened faster than many had anticipated.11
The forecast by Ajeya Cotra
The last shown forecast stems from the research by Ajeya Cotra, who works for the nonprofit Open Philanthropy.12 In 2020 she published a detailed and influential study asking when the world will see transformative AI. Her timeline is not based on surveys, but on the study of long-term trends in the computation used to train AI systems. I present and discuss the long-run trends in training computation in this companion article.
Cotra estimated that there is a 50% chance that a transformative AI system will become possible and affordable by the year 2050. This is her central estimate in her “median scenario.” Cotra emphasizes that there are substantial uncertainties around this median scenario, and also explored two other, more extreme, scenarios. The timelines for these two scenarios – her “most aggressive plausible” scenario and her “most conservative plausible” scenario – are also shown in the visualization. The span from 2040 to 2090 in Cotra’s “plausible” forecasts highlights that she believes that the uncertainty is large.
The visualization also shows that Cotra updated her forecast two years after its initial publication. In 2022 Cotra published an update in which she shortened her median timeline by a full ten years.13
It is important to note that the definitions of the AI systems in question differ very much across these various studies. For example, the system that Cotra speaks about would have a much more transformative impact on the world than the system that the Metaculus forecasters focus on. More details can be found in the appendix and within the respective studies.
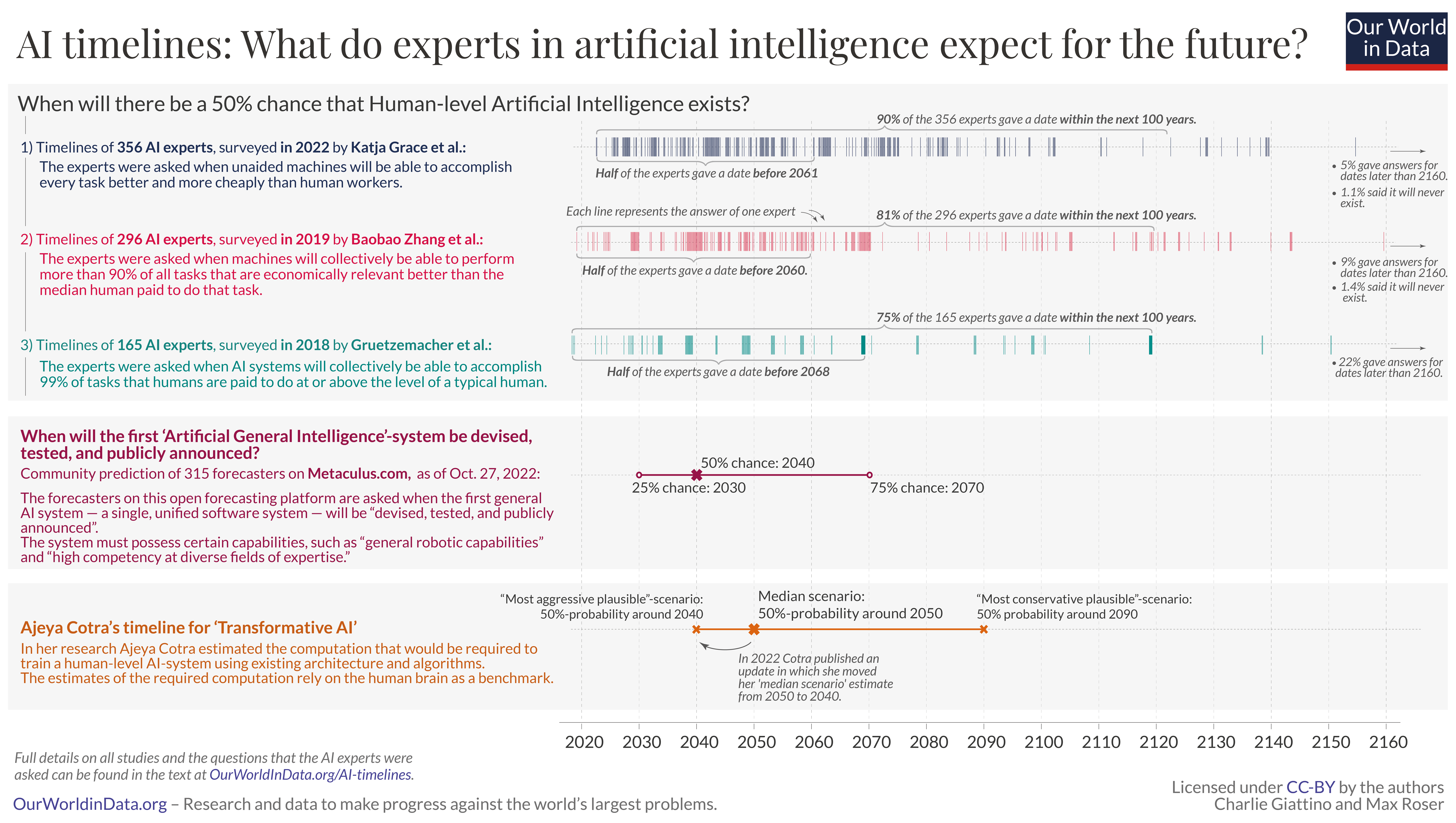
What can we learn from the forecasts?
The visualization shows the forecasts of 1128 people – 812 individual AI experts, the aggregated estimates of 315 forecasters from the Metaculus platform, and the findings of the detailed study by Ajeya Cotra.
There are two big takeaways from these forecasts on AI timelines:
- There is no consensus, and the uncertainty is high. There is huge disagreement between experts about when human-level AI will be developed. Some believe that it is decades away, while others think it is probable that such systems will be developed within the next few years or months.There is not just disagreement between experts; individual experts also emphasize the large uncertainty around their own individual estimate. As always when the uncertainty is high, it is important to stress that it cuts both ways. It might be very long until we see human-level AI, but it also means that we might have little time to prepare.
- At the same time, there is large agreement in the overall picture. The timelines of many experts are shorter than a century, and many have timelines that are substantially shorter than that. The majority of those who study this question believe that there is a 50% chance that transformative AI systems will be developed within the next 50 years. In this case it would plausibly be the biggest transformation in the lifetime of our children, or even in our own lifetime.
The public discourse and the decision-making at major institutions have not caught up with these prospects. In discussions on the future of our world – from the future of our climate, to the future of our economies, to the future of our political institutions – the prospect of transformative AI is rarely central to the conversation. Often it is not mentioned at all, not even in a footnote.
We seem to be in a situation where most people hardly think about the future of artificial intelligence, while the few who dedicate their attention to it find it plausible that one of the biggest transformations in humanity’s history is likely to happen within our lifetimes.
Acknowledgements: I would like to thank my colleagues Natasha Ahuja, Daniel Bachler, Bastian Herre, Edouard Mathieu, Esteban Ortiz-Ospina and Hannah Ritchie for their helpful comments to drafts of this essay.
And I would like to thank my colleague Charlie Giattino who calculated the timelines for individual experts based on the data from the three survey studies and supported the work on this essay. Charlie is also one of the authors of the cited study by Zhang et al. on timelines of AI experts.
More information about the studies and forecasts discussed in this essay
Endnotes
Peter Norvig and Stuart Russell (2021) – Artificial Intelligence: A Modern Approach. Fourth edition. Published by Pearson.
A total of 4,271 AI experts were contacted; 738 responded (a 17% rate), of which 352 provided complete answers to the human-level AI question.It’s possible that the respondents were not representative of all the AI experts contacted – that is, that there was “sample bias.” There is not enough data to rule out all potential sources of sample bias. After all, we don’t know what the people who didn’t respond to the survey, or others who weren’t even contacted, believe about AI. However, there is evidence from similar surveys to suggest that at least some potential sources of bias are minimal.
In similar surveys (e.g., Zhang et al. 2022; Grace et al. 2018), the researchers compared the group of respondents with a randomly selected, similarly sized group of non-respondents to see if they differed on measurable demographic characteristics, such as where they were educated, their gender, how many citations they had, years in the field, etc.
In these similar surveys, the researchers found some differences between the respondents and non-respondents, but they were small. So while other, unmeasured sources of sample bias couldn’t be ruled out, large bias due to the demographic characteristics that were measured could be ruled out.
Much of the literature on AI timelines focuses on the 50% probability threshold. I think it would be valuable if this literature would additionally also focus on higher thresholds, say a probability of 80% for the development of a particular technology. In future updates of this article we will aim to broaden the focus and include such higher thresholds.
A discussion of the two most widely used concepts for thinking about the future of powerful AI systems – human-level AI and transformative AI – can be found in this companion article.
The visualization shows when individual experts gave a 50% chance of human-level machine intelligence. The surveys also include data on when these experts gave much lower chances (e.g., ~10%) as well as much higher ones (~90%), and the spread between the respective dates is often considerable, expressing the AI experts range of their individual uncertainty. For example, the average across individual experts in the Zhang et al study gave a 10% chance of human-level machine intelligence by 2035, a 50% chance by 2060, and a 90% chance by 2105.
Mellers, B., Tetlock, P., & Arkes, H. R. (2019). Forecasting tournaments, epistemic humility and attitude depolarization. Cognition, 188, 19-26.
Tetlock, P. (2005) – Expert political judgment: How good is it? How can we know? Princeton, NJ: Princeton University Press
Philip E. Tetlock and Dan Gardner (2015) – Superforecasting: The Art and Science of Prediction.
Another example is Ernest Rutherford, father of nuclear physics, calling the possibility of harnessing nuclear energy "moonshine." The research paper by John Jenkin discusses why. John G. Jenkin (2011) – Atomic Energy is ‘‘Moonshine’’: What did Rutherford Really Mean?. Published in Physics in Perspective. DOI 10.1007/s00016-010-0038-1
This is discussed in some more detail for the study by Grace et al. in the Appendix.
See the previously cited literature on forecasting by Barbara Mellers, Phil Tetlock, and others.
There are two other relevant questions on Metaculus. The first one asks for the date when weakly General AI will be publicly known. And the second one is asking for the probability of ‘human/machine intelligence parity’ by 2040.
Metaculus’s community prediction fell from the year 2058 in March 2022 to the year 2040 in July 2022.
Her research was announced in various places, including the AI Alignment Forum: Ajeya Cotra (2020) – Draft report on AI timelines. As far as I know the report itself always remained a ‘draft report’ and was published here on Google Docs.
In 2022 Ajeya Cotra published a Two-year update on my personal AI timelines.
Ajeya Cotra’s Two-year update on my personal AI timelines.
Grace et al (2018) Viewpoint: When Will AI Exceed Human Performance? Evidence from AI Experts. Journal of Artificial Intelligence Research. We read both of these numbers of the chart in this publication, these years are not directly reported.
Cite this work
Our articles and data visualizations rely on work from many different people and organizations. When citing this article, please also cite the underlying data sources. This article can be cited as:
Max Roser (2023) - “AI timelines: What do experts in artificial intelligence expect for the future?” Published online at OurWorldinData.org. Retrieved from: 'https://ourworldindata.org/ai-timelines' [Online Resource]
BibTeX citation
@article{owid-ai-timelines,
author = {Max Roser},
title = {AI timelines: What do experts in artificial intelligence expect for the future?},
journal = {Our World in Data},
year = {2023},
note = {https://ourworldindata.org/ai-timelines}
}
Reuse this work freely
All visualizations, data, and code produced by Our World in Data are completely open access under the Creative Commons BY license. You have the permission to use, distribute, and reproduce these in any medium, provided the source and authors are credited.
The data produced by third parties and made available by Our World in Data is subject to the license terms from the original third-party authors. We will always indicate the original source of the data in our documentation, so you should always check the license of any such third-party data before use and redistribution.
All of our charts can be embedded in any site.