How do researchers estimate the death toll caused by each risk factor, whether it’s smoking, obesity, or air pollution?
Risk factors are important to understand because they can help us identify how to save lives. How do researchers estimate their impact?
When someone dies, they are usually given a single underlying cause – a particular disease or injury – on their death certificate. But beneath each disease or injury, there can be a range of factors that make the disease or injury more likely to occur.
These are called risk factors. We’re all familiar with many: smoking is a risk factor for lung cancer1, lack of exercise is a risk factor for heart disease, and air pollution is a risk factor for respiratory diseases.
Understanding these factors is important because they can help us identify how to reduce disease and death.
In this article, I explain what a risk factor is, how researchers estimate how many deaths are caused by risk factors, and how to interpret these estimates.
What is a risk factor?
By its simplest definition, a risk factor is a characteristic that predicts a negative health outcome to a meaningful degree.2
Let's use the example of lung cancer. A risk factor of lung cancer is a characteristic that is correlated with someone having a meaningfully higher risk of developing lung cancer later on.
But the term is much more valuable when it is used to describe a causal risk factor – something that predicts an outcome because it has an effect on the outcome and affects the chance that it will occur.
In this case, a risk factor of lung cancer would increase the chances that someone develops the disease.
The difference between these two usages of the term is essential.
For example, people who drink coffee frequently are more likely to develop lung cancer than those who do not. But this is not because drinking coffee causes lung cancer; it is because people who drink coffee frequently are also more likely to be smokers, and smoking increases the risk of lung cancer.3
So, by the first definition of a risk factor – a characteristic that simply predicts an outcome – both drinking coffee frequently and smoking may be described as risk factors for lung cancer.
But, by the second definition – a causal risk factor, which increases the chances of the outcome – smoking would be considered a risk factor for lung cancer, but drinking coffee would not.
Identifying causal risk factors has more benefits to public health because, with the knowledge that a risk factor is causal, we can better understand how to reduce its consequences.
Knowing that smoking causes lung cancer guided public health campaigns to focus on smoking cessation, regulation, and substitutes, reducing the rate of lung cancer globally.
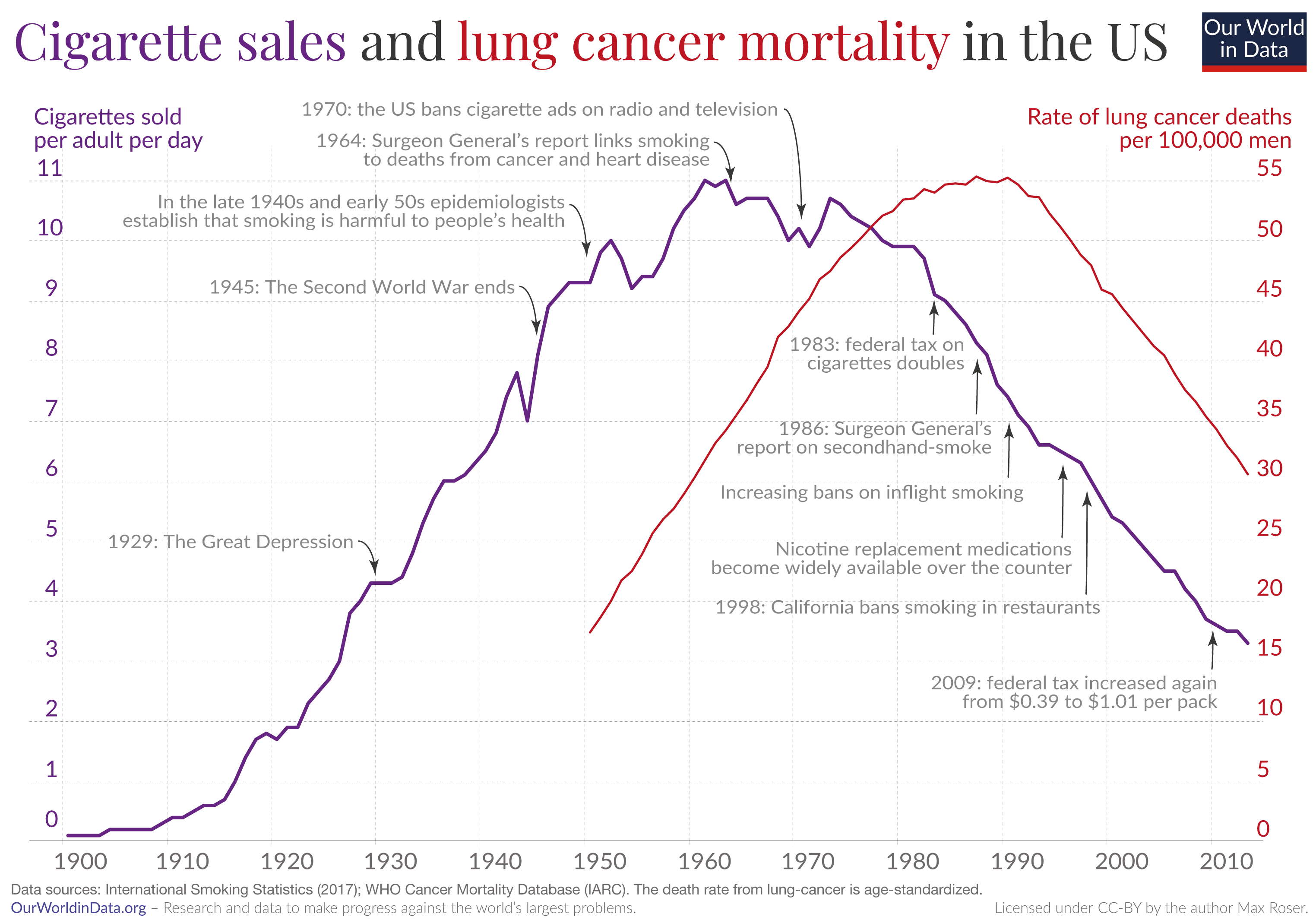
How do researchers know if a risk factor is causal?
To understand whether a risk factor causes an outcome, researchers can look at different sources of evidence.
For example, it was clear as early as the 1960s that the large increase in lung cancer rates worldwide was caused by the increase in smoking.4
Animal experiments, biopsies, and population studies all showed that cigarette smoke was associated with specific changes in lung tissue and lung cancer incidence. The association was so large and consistent that other risk factors could not explain it, and scientists were confident that it was a causal risk factor for lung cancer.5
Randomized controlled trials (RCTs) are often a key source of evidence to identify causal risk factors. These are experiments where people are given, at random, either an intervention (such as a drug) or a control (such as a placebo pill) and followed up to see how they fare on various outcomes.
Through RCTs, researchers can learn about the effects of risk factors by reducing the risk factor and monitoring whether it reduces the incidence of the disease.
For example, we know influenza increases the risk of cardiovascular disease because flu vaccines reduce the risk of cardiovascular disease. This has been demonstrated in RCTs where people were given flu vaccines or not, and followed up for several years.6
In this article on Our World in Data, I have written more about Randomized Controlled Trials:
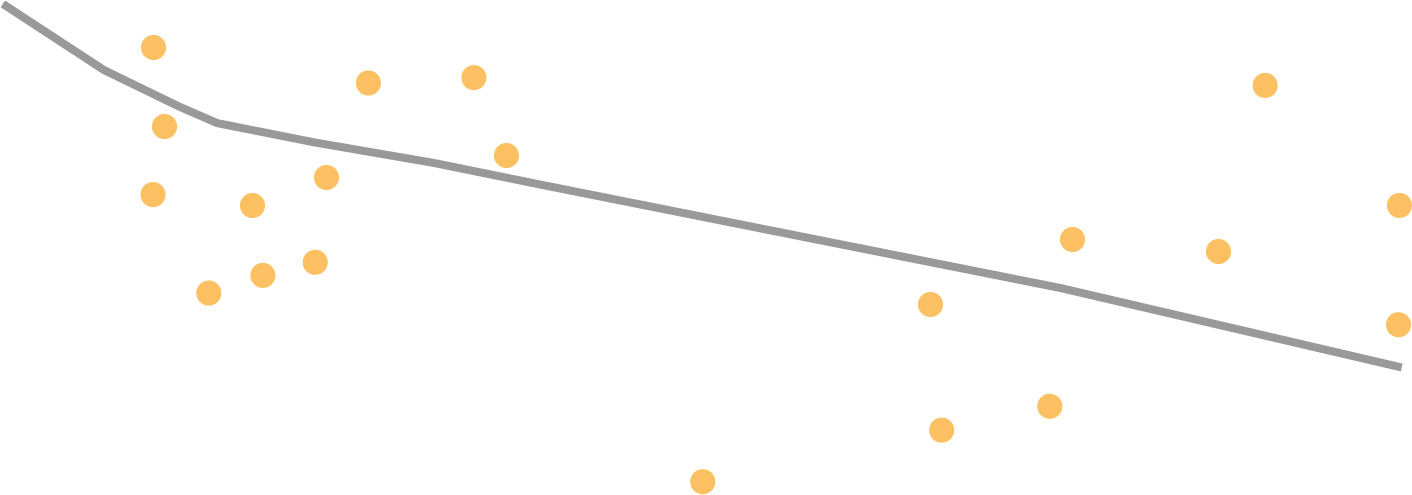
Why randomized controlled trials matter and the procedures that strengthen them
How do researchers estimate how many people die from a causal risk factor?
Below are two approaches to estimating how many people die from a risk factor. Each of these approaches has different strengths and limitations.
Estimating deaths that could have been prevented
One popular measure is the ‘population-attributable fraction’, which is an estimate of the fraction of deaths caused by a risk factor.7
It is an estimate of the number of deaths that would be prevented if the risk factor was eliminated (for factors such as smoking) or reduced to an optimal level (for example, when looking at obesity, researchers may estimate the deaths prevented if the body-mass index (BMI) in the population was reduced to an ‘optimum’ level).
To do this, researchers use estimates of the prevalence of the risk factor and how much it increases the risk of dying.
Let’s take smoking as an example. Researchers know from many studies that smoking increases the risk of death from cancers, heart disease, diabetes, tuberculosis, and other causes of death. But how many deaths does it cause?
In the chart, you can see an example using data from the United States.8
The chart shows how smoking increases the risk of death from various causes. It shows, for example, that male smokers have 21 times the risk of dying from lung cancer than those who have never smoked.
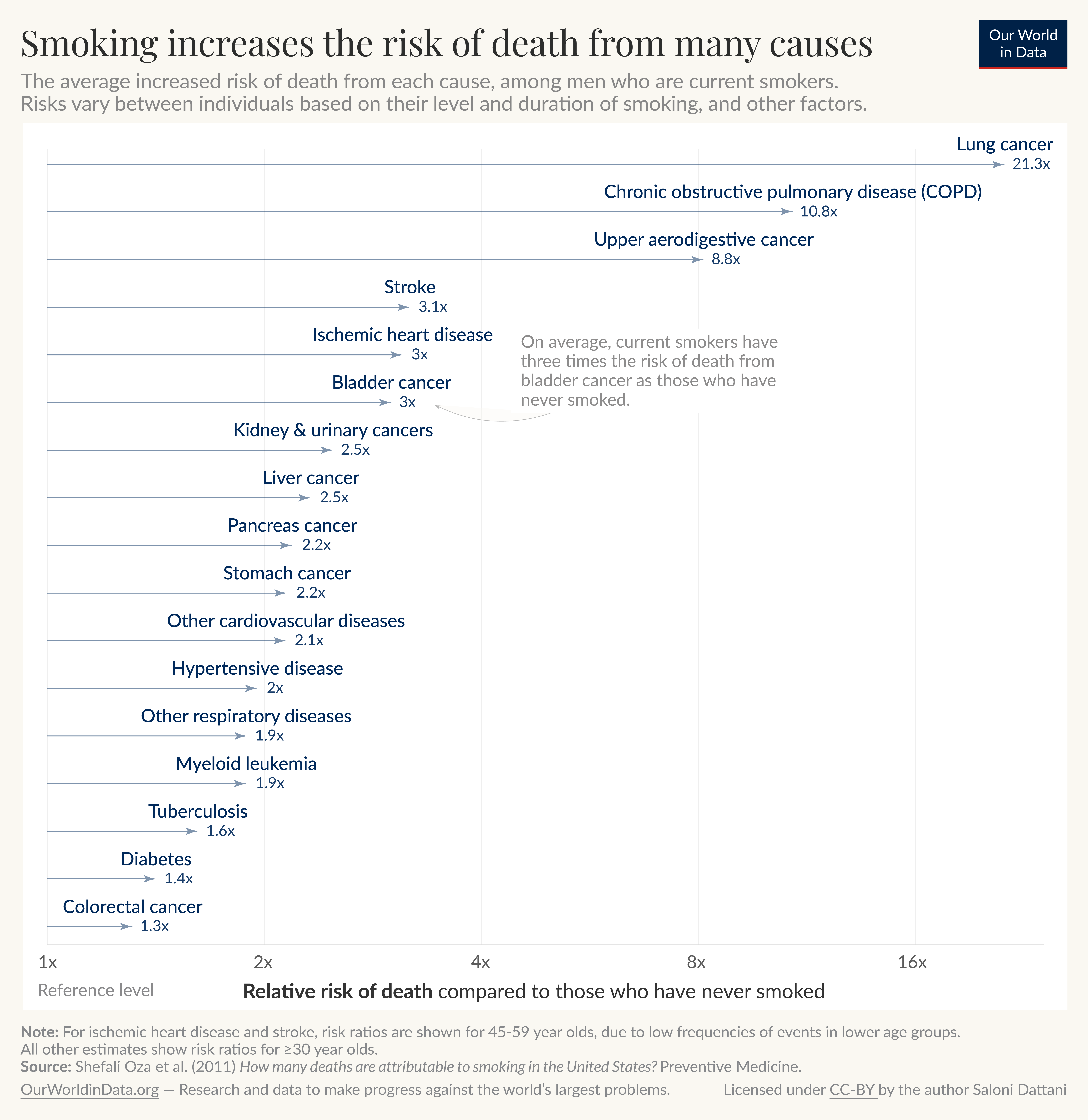
Using these estimates and the fraction of people who are smokers, researchers can estimate the number of deaths caused by smoking.
This approach can be useful for estimating deaths caused by an ongoing risk factor, especially when there are robust estimates of how much the risk factor increases the risk of death.
However, there are several important things to keep in mind.
First, the method depends on having a good estimate of how much the risk factor increases the risk of death.
To do this, it’s important to have good data on the causes of death from death certificates, epidemiological research to estimate the impact of risk factors on death, and data on the prevalence of these risk factors in the general population.
In the example of smoking, data on smoking prevalence comes from national surveys – but people tend to underreport their smoking behaviors. This means that many smokers would be labeled as non-smokers, leading to underestimating the number of deaths caused by smoking.
A related problem is that the risk ratio can vary between people. For example, the category ‘current smoker’ is very broad, as people have smoked for different lengths of time.
A third problem is that other confounding factors can be present. For example, smokers may also have other risk factors or behaviors that increase their risk of death. In the example above, the researchers had adjusted for other known confounding factors, but this is not always simple.
In the chart below, you can see the Institute for Health Metrics Evaluation (IHME) estimates of the global number of deaths caused by each risk factor.9
It shows that in 2019, almost 11 million people died as a result of high blood pressure, and almost 8 million died as a result of smoking. Many other risk factors were also estimated to have caused many deaths.
It’s important to note that the risk factors are not exclusive – people could be exposed to multiple risk factors simultaneously – and the number of deaths from individual risk factors does not sum to the total number of deaths.
In this related article, I explain why:
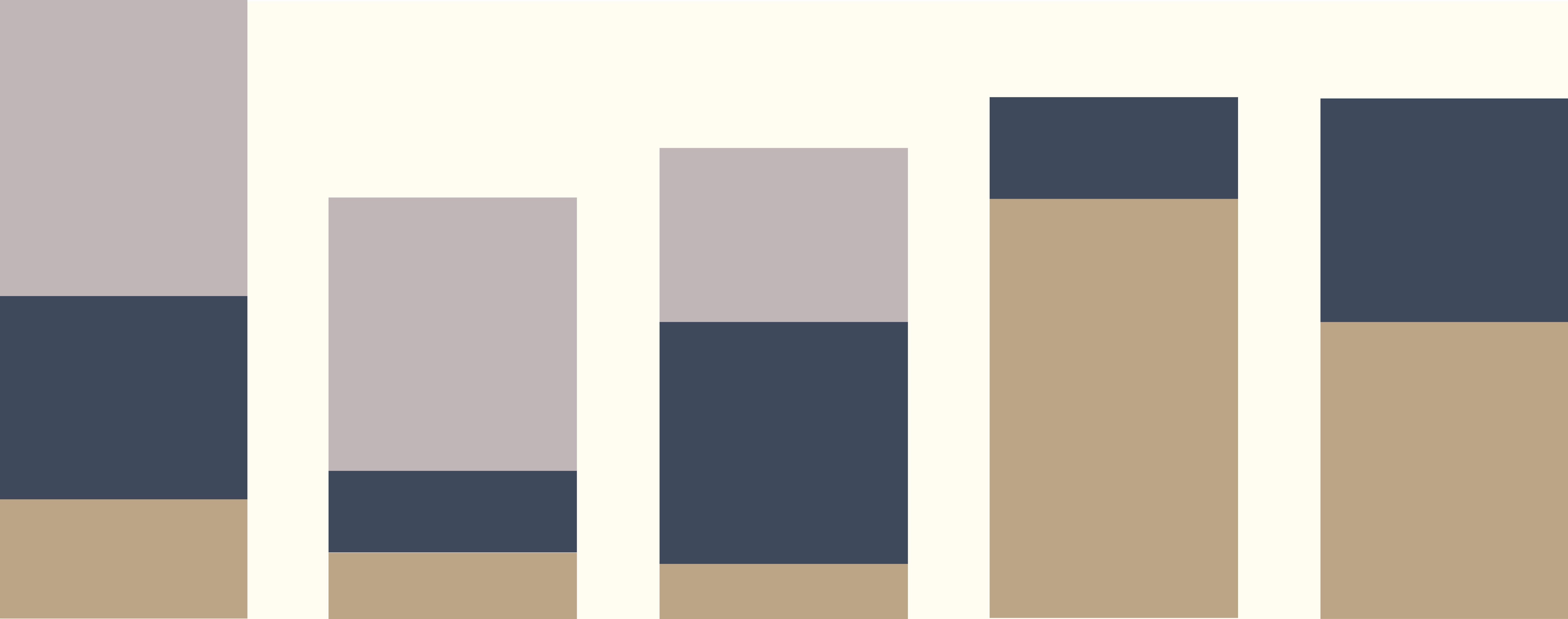
Why isn’t it possible to sum up the deaths from different risk factors?
Estimating the death toll of risk factors with excess deaths
Another approach to estimating the number of people who die from a causal risk factor relies on statistics of excess deaths.
This method estimates the additional number of deaths that occur when a risk factor is present – compared to a baseline.
This can be useful when the risk factor is a new or external event that affects the population, such as a heatwave, natural disaster, or new infectious disease.
For example, researchers can estimate the number of excess deaths caused by a heatwave by comparing the number of deaths during the heatwave to a baseline figure.
To do this, the researchers must identify when the risk factor affected the population.
For example, this chart comes from a study that estimated the number of deaths across European countries during the 2022 summer heat wave.10
The researchers looked at the number of weekly deaths in 2022 and how it compared to the baseline between 2015 and 2022. They used high-resolution temperature data from each region.
Through this method, they estimated an excess of around 63,000 heatwave deaths across Europe in 2022.
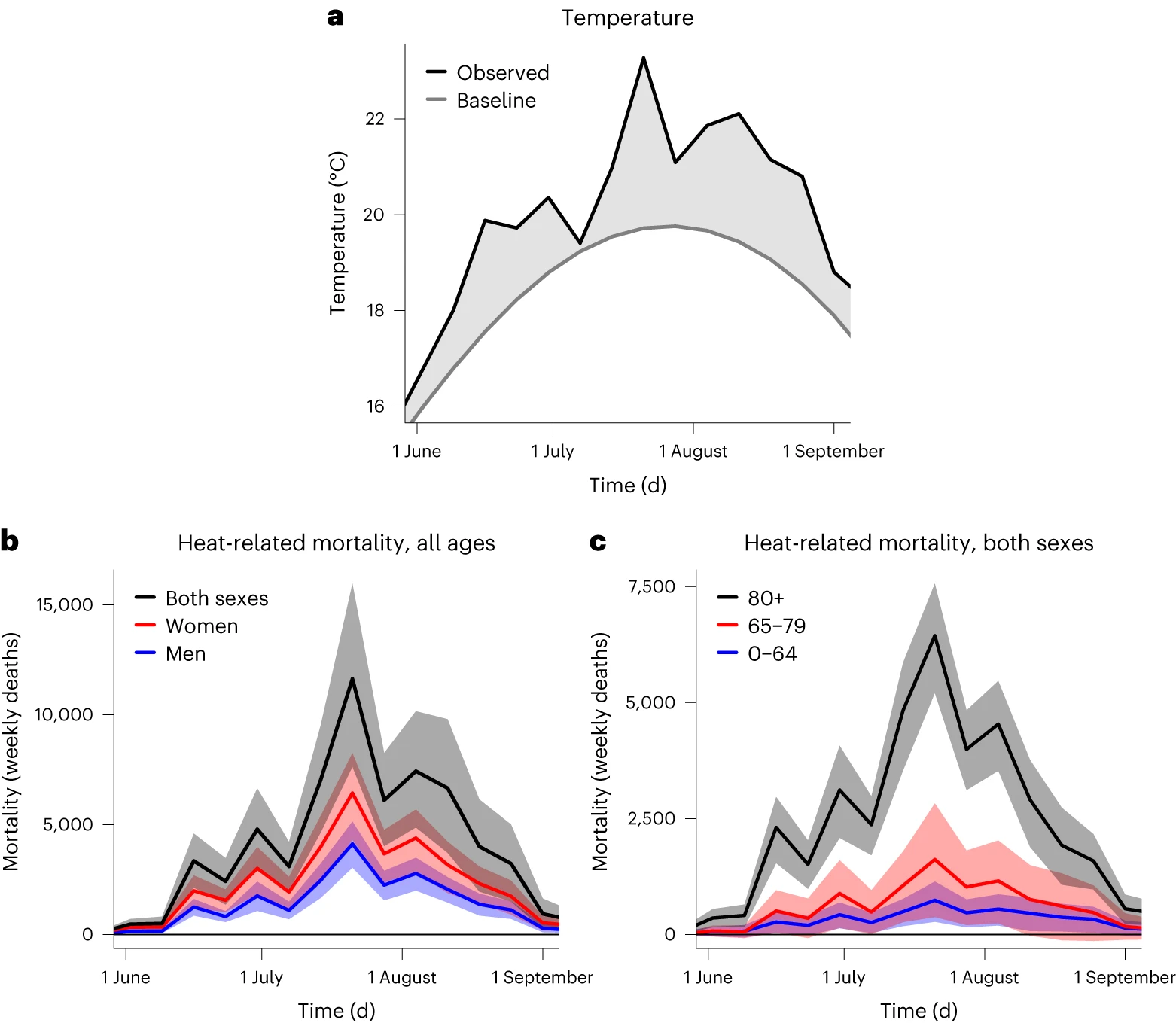
With an excess death approach, researchers can try to understand the overall impact of the risk factor – even if the risk factor causes death in different ways or if the cause of death is inaccurate or missing from death certificates.
But it’s important to remember that deaths estimated through this method are not necessarily specific to the risk factor.
Instead, it estimates the net change compared to the baseline expected number of deaths. However, there may be other risk factors affecting the number of deaths simultaneously, for example, another disease outbreak, disaster, air pollution, or changes in other behavior or trends.11
Conclusion
Researchers use different methods to estimate the deaths caused by each risk factor, but these have important strengths and limitations.
They depend on good underlying death records, epidemiological studies on the causal effects of risk factors, and the prevalence of these risk factors in the population.
Although it may sound simple to attribute a person’s death to a risk factor, this is usually not straightforward. People’s chances of dying are affected by various risk factors over their lifetimes. This also means the same deaths can be prevented in multiple ways.
By estimating the effects of different risk factors and how many deaths they cause, we can identify better ways to save lives.
Acknowledgements
Edouard Mathieu, Hannah Ritchie, and Max Roser provided valuable feedback on this article.
Endnotes
Some behaviors – such as substance abuse (including alcoholism and chronic smoking) – are usually thought of as risk factors, but they also have ICD death codes. Doctors can list them on the death certificate as the underlying cause of death if they think this is the case.
Brooks, E. G., & Reed, K. D. (2015). Principles and Pitfalls: A Guide to Death Certification. Clinical Medicine & Research, 13(2), 74–82. https://doi.org/10.3121/cmr.2015.1276
But doctors may not be certain about how much each risk factor contributed to their death.
Kraemer, H. C., Kazdin, A. E., Offord, D. R., Kessler, R. C., Jensen, P. S., & Kupfer, D. J. (1997). Coming to terms with the terms of risk. Archives of General Psychiatry, 54(4), 337–343.Kindig, D. A. (2007). Understanding Population Health Terminology: Understanding Population Health Terminology. Milbank Quarterly, 85(1), 139–161. https://doi.org/10.1111/j.1468-0009.2007.00479.x
Galarraga, V., & Boffetta, P. (2016). Coffee Drinking and Risk of Lung Cancer—A Meta-Analysis. Cancer Epidemiology, Biomarkers & Prevention, 25(6), 951–957. https://doi.org/10.1158/1055-9965.EPI-15-0727
Hill, G., Millar, W., & Connelly, J. (2003). “The Great Debate”: Smoking, Lung Cancer, and Cancer Epidemiology. Canadian Bulletin of Medical History, 20(2), 367-386.
Cornfield, J., Haenszel, W., Hammond, E. C., Lilienfeld, A. M., Shimkin, M. B., & Wynder, E. L. (1959). Smoking and Lung Cancer: Recent Evidence and a Discussion of Some Questions. JNCI: Journal of the National Cancer Institute. https://doi.org/10.1093/jnci/22.1.173
Behrouzi, B., Bhatt, D. L., Cannon, C. P., Vardeny, O., Lee, D. S., Solomon, S. D., & Udell, J. A. (2022). Association of Influenza Vaccination With Cardiovascular Risk: A Meta-analysis. JAMA Network Open, 5(4), e228873. https://doi.org/10.1001/jamanetworkopen.2022.8873
Poole, C. (2015). A history of the population attributable fraction and related measures. Annals of Epidemiology, 25(3), 147–154. https://doi.org/10.1016/j.annepidem.2014.11.015
Oza, S., Thun, M. J., Henley, S. J., Lopez, A. D., & Ezzati, M. (2011). How many deaths are attributable to smoking in the United States? Comparison of methods for estimating smoking-attributable mortality when smoking prevalence changes. Preventive Medicine, 52(6), 428–433. https://doi.org/10.1016/j.ypmed.2011.04.007
Murray, C. J. L., Aravkin, A. Y., Zheng, P., Abbafati, C., Abbas, K. M., Abbasi-Kangevari, M., Abd-Allah, F., Abdelalim, A., Abdollahi, M., Abdollahpour, I., Abegaz, K. H., Abolhassani, H., Aboyans, V., Abreu, L. G., Abrigo, M. R. M., Abualhasan, A., Abu-Raddad, L. J., Abushouk, A. I., Adabi, M., … Lim, S. S. (2020). Global burden of 87 risk factors in 204 countries and territories, 1990–2019: A systematic analysis for the Global Burden of Disease Study 2019. The Lancet, 396(10258), 1223–1249. https://doi.org/10.1016/S0140-6736(20)30752-2
These estimates adjust for the effects that are mediated by other risk factors.
Ballester, J., Quijal-Zamorano, M., Méndez Turrubiates, R. F., Pegenaute, F., Herrmann, F. R., Robine, J. M., Basagaña, X., Tonne, C., Antó, J. M., & Achebak, H. (2023). Heat-related mortality in Europe during the summer of 2022. Nature Medicine, 29(7), 1857–1866. https://doi.org/10.1038/s41591-023-02419-z
Another problem is that it can be difficult to decide the start and end date of the risk factor. Depending on how many days are counted as part of the heatwave, the number of excess deaths attributed to it can vary.
A final limitation of excess deaths is that it’s hard to estimate the baseline number of deaths that would have occurred during that time, without the risk factor, because of other long-term trends.
For example, the number of annual deaths can change over time due to an aging population and general improvements in health and living standards.
Without accounting for this long-term decline, which affects the baseline expected number of deaths, researchers may underestimate the number of deaths caused by a risk factor.
Cite this work
Our articles and data visualizations rely on work from many different people and organizations. When citing this article, please also cite the underlying data sources. This article can be cited as:
Saloni Dattani (2023) - “How do researchers estimate the death toll caused by each risk factor, whether it’s smoking, obesity, or air pollution?” Published online at OurWorldInData.org. Retrieved from: 'https://ourworldindata.org/how-do-researchers-estimate-the-death-toll-caused-by-each-risk-factor-whether-its-smoking-obesity-or-air-pollution' [Online Resource]
BibTeX citation
@article{owid-how-do-researchers-estimate-the-death-toll-caused-by-each-risk-factor-whether-its-smoking-obesity-or-air-pollution,
author = {Saloni Dattani},
title = {How do researchers estimate the death toll caused by each risk factor, whether it’s smoking, obesity, or air pollution?},
journal = {Our World in Data},
year = {2023},
note = {https://ourworldindata.org/how-do-researchers-estimate-the-death-toll-caused-by-each-risk-factor-whether-its-smoking-obesity-or-air-pollution}
}
Reuse this work freely
All visualizations, data, and code produced by Our World in Data are completely open access under the Creative Commons BY license. You have the permission to use, distribute, and reproduce these in any medium, provided the source and authors are credited.
The data produced by third parties and made available by Our World in Data is subject to the license terms from the original third-party authors. We will always indicate the original source of the data in our documentation, so you should always check the license of any such third-party data before use and redistribution.
All of our charts can be embedded in any site.